
2016-2018
iOS & Android
Role
Design & Research Lead
Expertise
Product Design
UI/UX
Design System
Research Lead
Strategy
Deliverables
Research Agenda
Research Insights
UX Flow
UI Screens
Prototypes
Capital One possesses extensive transaction data and aimed to discover novel, practical applications for it. Recognizing that food expenditures represent customers' most common transactions, we proposed employing machine learning to refine and discern patterns, potentially enhancing dining suggestions and boosting spending within this sector.
Problem
Proceeding directly to the creation of a customer-centric Minimum Viable Product (MVP) entailed substantial risks. Prior to allocating the necessary resources and time for developing an effective restaurant recommendation platform, we had to confirm the viability of three key elements.
Execution
Proceeding directly to the creation of a customer-centric Minimum Viable Product (MVP) entailed substantial risks. Prior to allocating the necessary resources and time for developing an effective restaurant recommendation platform, we had to confirm the viability of three key elements.
1
Tech POC
Prove this data can be used for a product like this
2
Product MVP
Prove data can deliver better recs than competition
3
Customer Pilot
Deliver transaction powered recs for all customers
Final Product
Results
The product proved to be successful. However, given the unresolved discovery problem and the significant costs associated with maintaining the data, the decision was made to shelve the project temporarily. We plan to revisit the initiative in the future when the requisite resources and funding become available.
NPS & Brand
Customers who utilized the Dining Explorer feature awarded a 5% increase in Net Promoter Score (NPS).
Session Length
Increased by approximately 20 seconds per user, the restaurant details page emerging as the most frequently viewed feature within the application.
Spend
On average, customers who utilized Dining Companion spent $200 more per month than non-users of the dining feature.
Discovery
Despite the quality of our recommendations, customers remained hesitant accessing restaurant suggestions within a banking application. At the time, the company was not prepared to launch a standalone application, leaving us with no immediate resolution.
Session Count
On average, the session count for Dining Explorer users within the Capital One application increased by 6 per month.
1
Before developing a customer-facing product, we ensured data feasibility and user satisfaction. We created a machine learning model to generate three text files with varying results: popular, trending, and local favorites. I then led a research study with 20 dining experts nationwide to evaluate unfamiliar top-ranking restaurants.
Research Confirmed Success
Based on the initial test and minimal effort invested, we discovered that our lists effectively identified top dining establishments. Moreover, they revealed previously unknown venues that customers have since become avid supporters of. This provided us with the necessary confidence and evidence to further invest in this concept, allowing us to begin prototyping our inaugural user experience as we progress toward the development of a Minimum Viable Product (MVP).
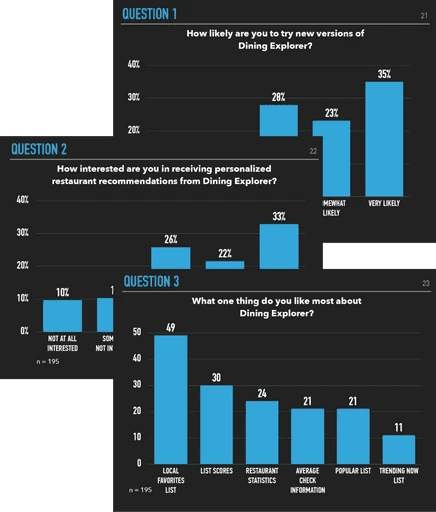
2
Design Jam
Leveraging the recently acquired research data, I guided the team through a design jam session. We outlined a storyboard detailing the product's usage and timeline, generating a multitude of ideas regarding its appearance and features. Following a thorough refinement process to align with the project scope and testing plan, we developed a prototype, enabling us to evaluate variations and commence the construction of a tangible product.
Product MVP
Drawing on the insights gained from our research, the team designed a streamlined three-page flow, featuring an entry banner, search page, and list view. By implementing this approach, we were able to launch the product in the market and collect quantitative data from thousands of users across the nation.
Recs Worked
The utilization of individual user data to refine restaurant recommendations proved to be remarkably successful. Users discovered previously unknown dining options and enthusiastically shared their positive experiences with others.
Planning Travel
Users were predominantly searching for dining options in locations they intended to visit, suggesting the potential for creating travel lists and guiding users through a city during their visit.
Discovery Issues
Integrating the feature within the Capital One banking application caused confusion among users, as they were primarily focused on financial management tasks, leading to a lack of interest in perusing food recs.
A Lot of Filtering
Customers exhibited a preference for filtering search results based on location, cuisine, and mealtime characteristics, investing significantly more time in these activities than in any other filtering options.
3
Location
Given the significant number of users employing the application to plan trips, we strategically prioritized location, utilizing it as the theme for the entire page.
Multi-Lists
Users reported discovering previously unknown dining options, which they enjoyed. The latest design boasts several personalized lists, highlighting cuisines, mealtime preferences, and unique local data.
New Style
As this marked our full-featured product launch, we constructed a new design system, emphasizing compelling imagery, bold headlines, and streamlined filters that eliminate the need for menu access.
Time of Day
We reoriented our focus towards using mealtime as the primary theme for our recs. This strategy enabled users to bypass filtering while providing greater flexibility for more specific searches, as needed.